Humans are so good at recognizing images because right from the day we are born, our eyes are constantly taking pictures of the environment around us and our brain is processing these images and making meaning out of these images. If we assume that our eyes take around one picture per second, a ten-year-old kid would have processed around 315,360,000 images.
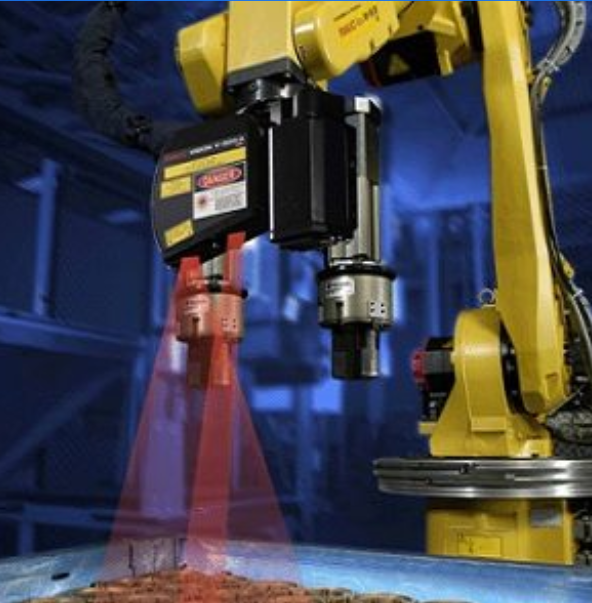
To convert images into a machine-readable format, certain algorithms are used to process the images. One of these algorithms is the Difference of Gaussians algorithm (DoG). This process helps in detecting the edges and outlines of the image.
In this article, we will try to give a simple to understand overview of how computer vision systems work. Machines are much better than humans at certain tasks such as computation, searching for text within a huge database and more. When it comes to performing simple tasks such as recognizing a cat or a tree in an image, computers fail miserably.
People are typically confused regarding what machine vision will and can’t do for a producing line or method. Understanding however it works will facilitate create selections regarding if it’ll resolve issues with the appliance. thus specifically what’s machine vision, and the way will it work?
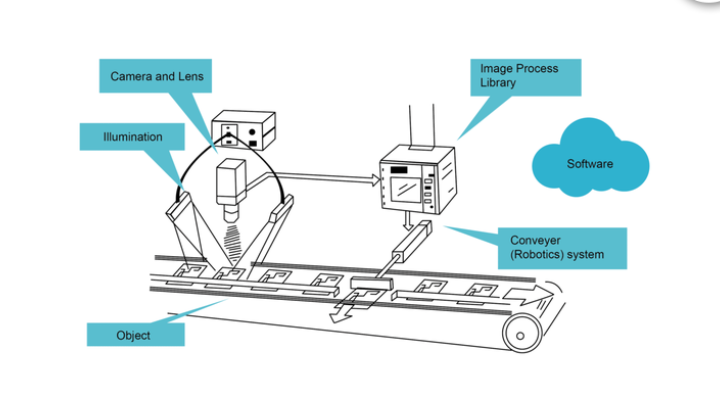
Machine vision is the use of a camera or multiple cameras to examine and analyze objects mechanically, typically an associate industrial or production setting. the information noninheritable then will be accustomed to managing a method or producing activity. A typical application can be on the associate assembly line; when the associate operation is performed on a neighborhood, the camera is triggered to capture and method a picture. The camera is also programmed to ascertain the position of one thing, its color, size or form, or whether or not the thing is there or not. It can also inspect and decipher a typical or 2-D matrix bar code or perhaps browse written characters.
After the merchandise has been inspected, an indication is typically generated to work out what to try and do with it. The half can be rejected into instrumentation or associate outcome conveyor or passed on through a lot of assembly operations, and chase its review results through the system. In any case, machine vision systems will give heaps more data regarding associate objects than easy absence/presence sort sensors.
Typical uses for machine vision include:
Quality assurance
Robot/machine steerage
Test and activity
Real-time method management
Data assortment
Machine observation
Sorting/counting.
Many makers use machine-driven machine vision rather than human inspectors as a result of it’s higher suited to repetitive review tasks. it’s quicker, a lot of objectives, and works endlessly. Machine vision systems will examine lots of or perhaps thousands of elements per minute, and provide a lot of consistent and reliable review results than human inspectors.
By reducing defects, increasing yield, facilitating compliance with rules and chasing elements with machine vision, makers will economize and increase gain.’
Machine vision is used in various industrial and medical applications. Examples include:
- Electronic component analysis
- Signature identification
- Optical character recognition
- Handwriting recognition
- Object recognition
- Pattern recognition
- Materials inspection
- Currency inspection
- Medical image analysis
How exactly does ‘Machine vision’ and ‘Computer vision’ differ?
Machine learning and computer vision are closely related. Computer vision uses techniques from machine learning and, in turn, some machine learning techniques are developed especially for computer vision.
The main difference is in focus: machine learning is more broad, unified not by any particular task but by similar techniques and approaches. Many machine learning algorithms and systems are pretty agnostic to what the machine is working on — you just need to provide the right set of inputs and features to the algorithm. Very similar classifiers can be used to block spam or identify pictures of cats.
Computer vision, on the other hand, is unified by a set of tasks: dealing with images. This requires a fair amount of different technologies — a fair bit of machine learning, to be sure, but also things from AI and signals processing and other fields.
Since the two fields share techniques and applications, they are pretty close. Chances are someone working in computer vision who also has a fair amount of experience with machine learning, and somebody in machine learning has at least some exposure to computer vision. (On the other hand, you wouldn’t expect either one to have any experience with programming language theory, for example.)
Human-computer interaction (HCI), on the other hand, is completely unrelated to these two fields. It’s the study of user interfaces. While there are certainly HCI projects that make use of computer vision or machine learning, these do not get featured any more than other CS sub-fields. If anything, HCI has more in common with psychology, industrial design or even marketing.
ADVANTAGES
- The machine vision system works faster than manual systems.
- The machine vision is consistent.
- Perform beyond human vision
- High repeatable in a controlled environment.
- No fatigue-24/7
- Reliable
- Good for unsafe and hazardous environment
- Operate well in space-constrained environments.
Machine vision in the automotive industry
If you have the chance to design the whole traffic system from scratch, you can probably do something very interesting.
For example, you can put electronic sensors along the roads and add communication chips to each car, so every car knows where every other car is and knows where exactly it is on the street. Traffic signals can also be enhanced with an RF chip, so they can reliably tell every car if it needs to stop or not. GPS can be combined with roadside sensors to better estimate car positions.
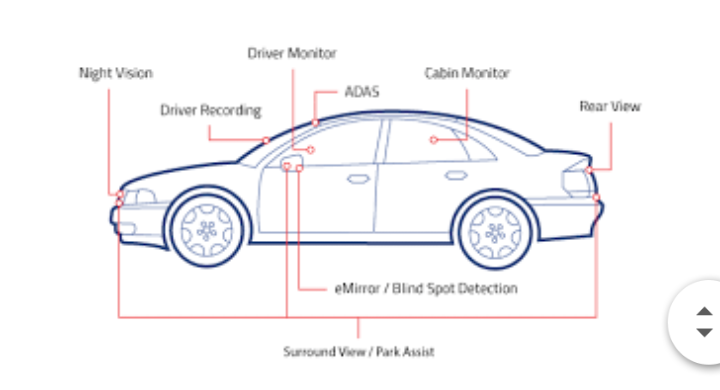
Such a system looks great and much more reliable than using computer vision techniques, however, it’ll be unlikely to happen in the near future.
Who’s gonna pay for all roadside sensors? Who’s gonna ensure that every car has a communication chip installed? What if an old manually driving car went rogue on the streets, how could other cars avoid a collision? If the sensors are malfunctioning, or a major power outage happens suddenly, what would happen?
So comes down to a conclusion, I believe computer vision techniques are irreplaceable on an autonomous car because it provides the last layer of security against unexpected incidents.
However, I always liked the sensor-based system described above. Maybe it would make sense to start with freeways. We can add sensors on the roadside, add a gate on on-ramps that filter out cars without communication chips, and notify the driver 5 minutes before the car arrives at the preset exit.
CONCLUSION
- Machine vision is less risky, reliable to use.
- It enables high-speed examination of defects in products
- The quality products can be obtained by the incorporation of machine vision systems.
Machine vision is the ability of a computer to see; it employs one or more video cameras, analog-to-digital conversion (ADC) and digital signal processing (DSP). The resulting data goes to a computer or robot controller. Machine vision is similar in complexity to voice recognition.
Machine vision systems rely on digital sensors protected inside industrial cameras with specialized optics to acquire images so that computer hardware and software can process, analyze, and measure various characteristics for decision making.
No comments:
Post a Comment